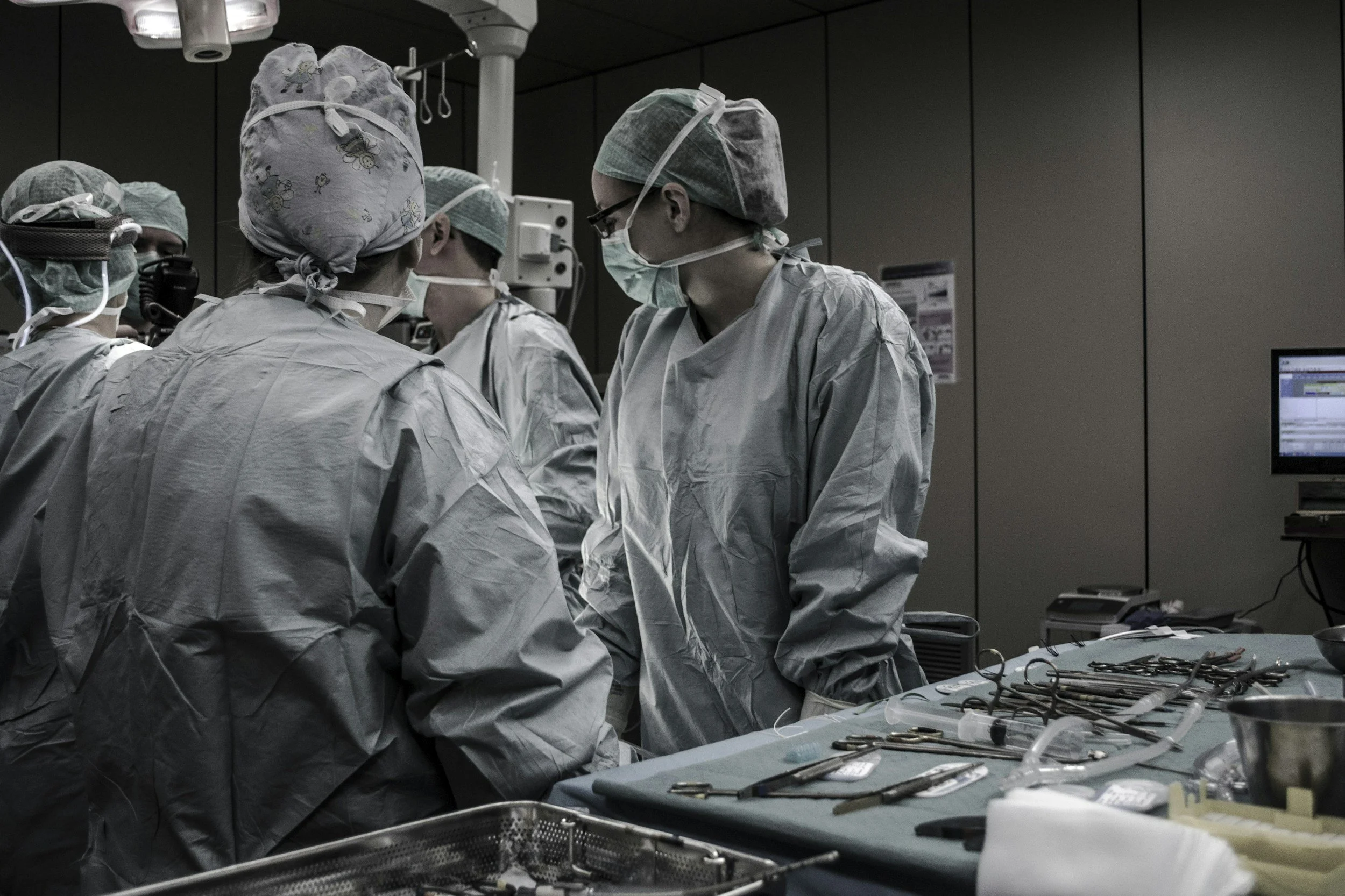
Using FHIR to Optimize Clinical Artificial Intelligence
I have to admit, I have a lot of #FOMO with the ubiquitous hype of artificial intelligence (AI). Especially its use in healthcare. It's exciting to see the variety of applications explored, notably those from large language model (LLM)-based, foundation models, like ambient scribes and semantic search, but I'm most intrigued with clinical reasoning. I'm also extremely skeptical of deploying them into production use cases in our healthcare systems from a safety and efficacy perspective.
Because of this, I've actually embarked on a bit of a side quest in 2024 to focus my efforts in diving deeper into Fast Healthcare Interoperability Resources (FHIR) because I think that it allows us to aggregate higher quality data from disparate systems that can be used to create benchmark datasets for improving these foundation models.
In this article, I want to explore a few thoughts I have about training, evaluating, and deploying clinical AI, with a focus on clinical reasoning, into production systems. For the purposes of this article, I use LLMs, foundation models, and clinical AI interchangeably with clinical AI focusing on the reasoning application.